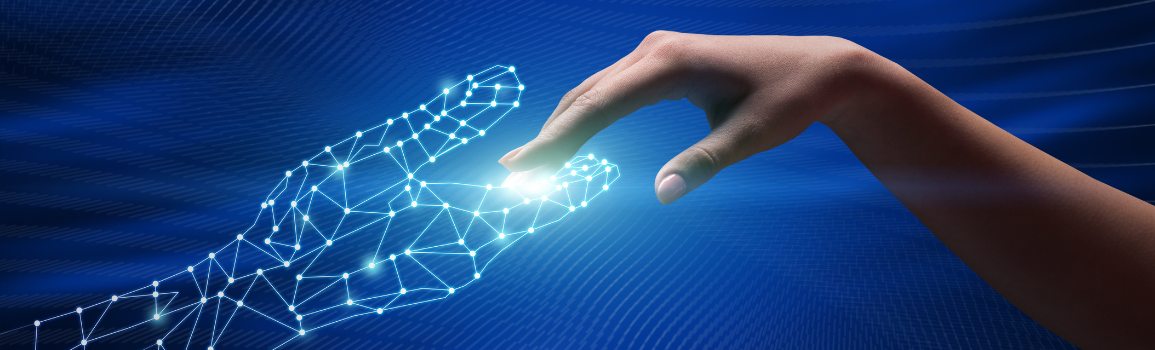
Generative artificial intelligence has the potential to fundamentally transform education and training. Thomas Friedman has argued that we are entering a new Promethean moment, when new tools, ways of thinking, or energy sources are introduced that are such an advance that they change how we work, how we learn–how we do everything. The arrival of generative AI tools such as ChatGPT has caused both anxiety and excitement among educators. On one hand, some have argued that they spell the end of writing assignments, and perhaps even the end of writing as a teachable skill. Some school systems, such as New York City schools, have gone so far as to ban ChatGPT from the classroom. Others have suggested that ChatGPT can take on a teaching role itself, for example teaching people how to code in Python.
Generative AI Has the Potential to Rapidly Scale Learning
I see great potential for generative AI to transform human learning, but only if it is used in the right way. This article presents some ways that generative AI can be used to transform learning. These insights are informed by learning science and extensive experience developing and delivering AI-enabled learning products. If applied properly, generative AI can accelerate learning and do so at scale, in ways that were never before possible. I also suggest some uses of generative AI to avoid, and how to guard against the risks of generative AI such as the risk that learners will use it to cheat and do their homework for them.
One of the strengths of generative AI chatbots is their ability to generate reasonable-sounding answers to just about any question, and do so very rapidly. If a learner is stuck and is looking for an answer to a particular question, this can be invaluable. If the learner wants further information, the chatbot can elaborate its explanation for them. And if it doesn’t generate the right answer, the learner can ask them again and get a different answer. Generative AI’s ability to generate a large number of responses quickly is quite remarkable.
Conversational Avatars Overcome Generative AI Shortcomings
At the same time, generative AI systems have shortcomings that can be significant in an educational context. The answers they provide are often excessively verbose, and they sometimes are inconsistent or just plain wrong. They sometimes “hallucinate” answers by combining various material found online. The OpenAI website is very clear about these limitations. This may not be a serious problem for experts and professionals who know the subject area and can recognize inappropriate answers, but novices can be easily misled by plausible-sounding but wrong answers.
Conversational avatars, in contrast, are a technology that has proven effective in educational settings and in employee upskilling and reskilling. Conversational avatars let learners practice their skills in spoken conversations. Avatars can play a variety of roles in simulation-based training, acting as simulated customers, co-workers, patients, and coaches. Avatars can also engage learners in Socratic dialogues. Instead of presenting multiple-choice questions and letting learners guess the answers, avatars can ask probing questions so that learners must respond in their own words and apply what they learned. This encourages problem-solving and critical thinking. Some educators are concerned that ChatGPT may undercut critical thinking and problem-solving skills; we find however that when designed properly AI-driven learning tools have just the opposite effect.
Avatar-based learning tools also benefit learners by encouraging them to practice, in a safe environment where there is no risk of embarrassment from making mistakes. Research shows that learning gains are a function of the number of times learners practice. Each time an avatar asks a question or elicits a response from a learner is a practice opportunity. Therefore, chatbots that answer questions are much less useful as learning tools than avatars that ask questions.
Spoken-language avatars are particularly useful because they reveal the learner’s degree of mastery of the material. Learners who respond rapidly and confidently have greater levels of mastery than learners who respond slowly and hesitantly. Also spoken-language avatars are resistant to cheating. If a learner tries to use ChatGPT to answer an avatar’s questions, it will take the learner a long time to respond and it will be very clear that the learner’s answers are not their own.
Alelo’s avatar-based learning systems have achieved significant results using this approach. In the XPRIZE Rapid Reskilling Competition, Alelo’s avatar-based training courses upskilled and reskilled community health workers at least twice as fast as conventional training and achieved retention rates that are double that of comparable online courses.
Generative AI Can Support Conversational Avatars and Other Learning Tools
Where I see the greatest potential for generative AI is not as learning tools per se but as generators of training data for other learning tools. To develop conversational AI, one needs training data–examples of dialogue utterances and responses. Generative AI can create such examples very quickly. A human expert is still needed to review the generated examples and weed out the “hallucinations” and other inappropriate responses. But reviewing and selecting generated examples is much quicker and easier than writing them from scratch. Then as learners interact with the avatars the learners’ responses are another source of training data. I also recommend giving users the option of marking avatar responses that they consider inappropriate or incorrect since that can inform the retraining process. Conversational avatars developed using this approach are more likely to interact appropriately with learners than chatbots trained on unfiltered Internet data.
Looking ahead, I see this approach as a way for instructional designers and subject matter experts to create their own instructional avatars. Instead of laboriously scripting avatar responses, they can rely on generative AI to generate candidate responses, and select the responses that they prefer.
Assuming a Support Role Mitigates the Risks of Generative AI
Generative AI technology continues to evolve very rapidly. GPT-4 and other tools have already emerged as successors to GPT-3. Yet meanwhile, many tech leaders have called for a moratorium on the development of the most advanced AI systems, so that their potential risks can be mitigated. AI-based tools that are informed by learning science and designed to promote learning do not pose the same risks. I believe that they will continue to have an advantage over general-purpose question-answering chatbots, even as the technology continues to develop.
As a final note: After I wrote this article I asked ChatGPT to write its own article on this topic. It produced a reasonable and coherent explanation of the potential benefits and risks of generative AI, and it made a suggestion that I thought was good and that I included in this article. But overall I found ChatGPT’s writing to be bland, not particularly insightful, and not sensitive to current trends in the field. I am glad that I chose to write this article myself, rather than rely on ChatGPT to write it for me.
RELATED: See my peer-reviewed journal article titled “How to Harness Generative AI to Accelerate Human Learning,” or learn more about bringing AI transformation to learning and development.
About The Author
Lewis Johnson
Dr. W. Lewis Johnson is President of Alelo and an internationally recognized expert in AI in education. He won DARPA’s Significant Technical Achievement Award and the I/ITSEC Serious Games Challenge, and was a finalist in XPRIZE Rapid Reskilling. He has been a past President of the International AI in Education Society, and was co-winner of the 2017 Autonomous Agents Influential Paper Award for his work in the field of pedagogical agents. He is regularly invited to speak at international conferences for distinguished organizations such as the National Science Foundation.