By Art Graesser, Ph.D.
Professor, Department of Psychology and the Institute of Intelligent Systems at the University of Memphis
Art Graesser will be presenting a webinar, Improving Comprehension Strategies of Struggling Adult Readers Through Conversational Trialogues With AutoTutor, on Wednesday, February 20, 1:00 PM-2:00 PM EDT / 10:00 AM-11:00 AM PDT and Thursday, February 21, 1:00 PM-2:00 PM EDT / 10:00 AM-11:00 AM PDT.
For many millennia, adult learning has primarily consisted of interactions with human mentors, tutors, teachers, and peers. During the last 25 years, these communicative actions and interactions have been simulated by computer agents in learning environments that have often shown significant learning gains.
As an example, my colleagues and I (https://sites.google.com/site/graesserart/) developed AutoTutor (https://link.springer.com/article/10.1007/s40593-015-0086-4, www.autotutor.org), a computer tutor that helps students learn by holding a conversation in natural language. AutoTutor does not perfectly understand the meaning of what the student verbally expresses (human tutors don’t either!). But AutoTutor understands the student well enough to generate questions, feedback, hints, and other dialogue moves that facilitate learning. Tutorial dialogue systems in the AutoTutor family have shown learning gains on many different subject matters, including computer literacy, physics, biology, math, and research ethics.
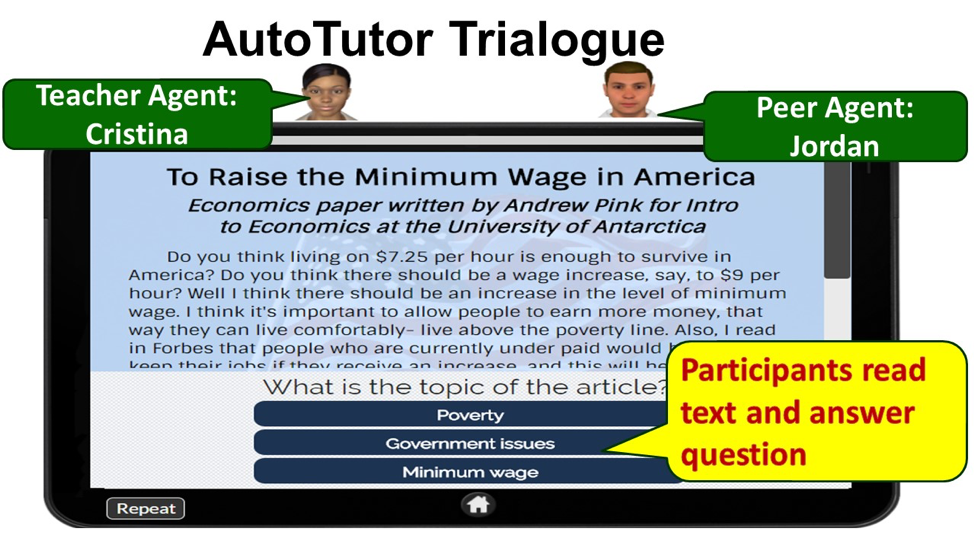
Sometimes it makes sense to add a second conversational agent to form a three-party conversation, what we call trialogues (https://files.eric.ed.gov/fulltext/ED586945.pdf), where a tutor agent and a peer agent interact with the learner. The two agents can model
AutoTutor has recently been developed to help struggling adult readers improve their comprehension strategies. One in six adults in the United States has low literacy skills and faces difficulties with daily literacy tasks. These concerns prompted the Institute of Education Sciences to fund the Center for the Study of Adult Literacy, a research center that focuses on adult literacy for adults who read between grades 3 to 8 (http://csal.gsu.edu). One objective of the Center was to develop technologies on the web (https://drive.google.com/file/d/1uNI68zj5CihAuk7TMhku0BsjFv06sqM0/view) that can be accessed by adults in literacy centers, their homes, and other locations. AutoTutor trialogues were developed to improve comprehension, as illustrated in the accompanying figure. Two agents interact with the adult as the text on minimum wages is read and the 3-alternative-choice question is answered. Cristina is the teacher agent and Jordan is the peer agent. The two agents guide the conversation by posing questions, giving feedback on incorrect responses of the human or peer agent, explaining why answers are correct or incorrect, and guiding adults who are lost using the interface. There are 35 AutoTutor lessons that target the theoretical levels of words, the meaning of the explicit text, the situation model with inferences, rhetorical structures, and digital technologies.
A recent study was conducted on 253 struggling adult readers in the Atlanta and Toronto areas. AutoTutor was included as part of an intervention that also included teacher-led instruction on word decoding, vocabulary, comprehension, and independent reading. Pretest and posttest scores assessed the impact of the intervention. We conducted data mining procedures that identified different clusters of readers based on their engagement and performance in the AutoTutor lessons (https://drive.google.com/file/d/1QRPXo34RZrFYtt0U4DFwa2lcNOclBF7P/view). Improvements were found on three different psychometric tests for most but not all clusters of readers. These results are guiding us in developing a new version of AutoTutor that is sensitive to the evolving performance profile of the struggling adult readers.
Art Graesser’s website with publications
https://sites.google.com/site/graesserart/
Graesser, A.C. (2016). Conversations with AutoTutor help students learn. International Journal of Artificial Intelligence in Education, 26, 124-132.
https://link.springer.com/article/10.1007/s40593-015-0086-4
Graesser, A.C., Forsyth, C., & Lehman, B. (2017). Two heads are better than one: Learning from agents in conversational trialogues. Teacher College Record, 119, 1-20.
https://files.eric.ed.gov/fulltext/ED586945.pdf
Graesser, A.C., Greenberg, D., Olney, A.M., & Lovett, M.W. (in press). Educational technologies that support reading comprehension for adults who have low literacy skills. In D. Perin (Ed). Wiley adult literacy handbook. New York: Wiley.
https://drive.google.com/file/d/1uNI68zj5CihAuk7TMhku0BsjFv06sqM0/view
Fang, Y., Shubeck, K.T., Lippert, A., Cheng,Q., Shi, G., Feng, S., Gatewood, J., Chen, S., Cai, Z., Pavlik, P. I., Frijters, J.C., Greenberg, D., Graesser, A. C. (2018). Clustering the learning patterns of adults with low literacy interacting with an intelligent tutoring system. In K.E. Boyer & M. Yudelson ( Eds.), Proceedings of the 11th International Conference on Educational Data Mining (pp.348-354). Buffalo, NY: Educational Data Mining Society.
https://drive.google.com/file/d/1QRPXo34RZrFYtt0U4DFwa2lcNOclBF7P/view